Prototyping
Interaction design
Design systems
Lifestyle management reimagined with the use of AI saving 2 hrs daily
Timeline
12 weeks (Jan 2024 - Apr 2024)
Team
UX Lead, UX Designer, UX Researcher
My role
UX and Project Lead
Tools
Figma, Zoom
In a nutshell
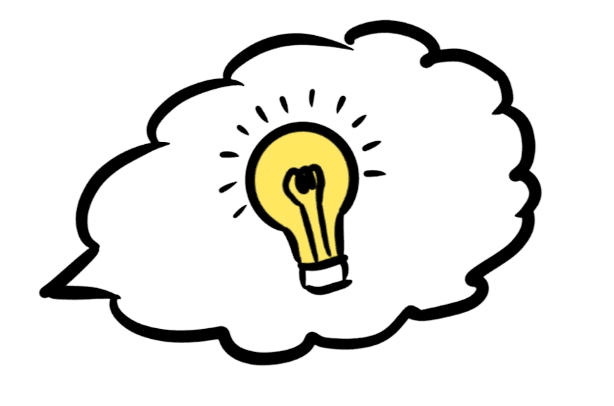
Conducted user interviews
Impact
9.2/10
satisfaction rating
2
hours saved daily
Problem space
Despite affecting millions, prediabetes is often overlooked!
On exploring the work done in the healthcare sector using AI, we realized that diabetes management was an emerging topic. On reading articles about diabetes management and statistics on diabetes, we noticed prediabetes - a health condition leading to diabetes which is often ignored, had very little research.
Research
For understanding the problems related to prediabetes and current research, we conducted a literature review followed by a competitive analysis. We later conducted interviews with 3 participants to understand the problem more deeply.
Literature review
Studied 15 research papers focusing on prediabetes management and role of AI in diabetes management.
We read a total of 15 research papers focusing on topics including diabetes management and AI, prediabetes management and methods of reversing prediabetes.
Competitive analysis
Studied 8 competitor apps based on fitness management or lifestyle management.
The team conducted a thorough competitive analysis of 8 applications in the domain of fitness or diabetes management. I studied 3 apps: LoseIt Calorie Counter, MyDiabetes and CaloScanAI.
Key insights
None of the 8 apps studied focused on prediabetes.
Most diabetes management apps focused on daily glucose monitoring - which is not a requirement for prediabetes
Regular fitness apps used for lifestyle management did not account for restrictions due to prediabetes.
The food scan feature was either for food or barcode and not both.
User research
Conducted interviews with 3 participants to understand their pain points.
To understand what people diagnosed with prediabetes do to manage their condition, we conducted interviews with three prediabetes patients.
Key insights
There was a lack of personalization in the currently existing apps.
Participants with no experience with using apps for prediabetes management expressed their concern with logging everything since it was a hastle and often got things wrong and said they couldn’t trust a smartphone app to manage their healthcare.
Problem definition
Brainstormed the pain points, defined objectives and designed a user flow for the solution.
After the thorough research, we brainstormed on identifying the major pain points that were not addressed.
Ideating the solution
The team performed a brainstorming session where we noted down our ideas on post-its and sorted them according to the requirements.
How might we
leverage AI to provide personalized meal and fitness recommendations, making prediabetes management easier and more efficient for users without overwhelming them?
Objectives
Personalized meal suggestions
Personalizing meal and exercise plans based on the user’s habits, goals and preferences.Make meal logging more efficient
Make scanning food easier and convenient for users to track.Allowing users to customize the recommendations
Making a system that learns from user input and enhances the personalization.
User flow
We then designed a user flow that captured all aspects of the features we aimed to include.
Design
Wireframes
Designed wireframes to represent the information architecture
With the flow designed, we started designing wireframes to establish a clear understanding of the app’s flow and structure.
Visual design
Followed Apple's design guidelines and created a design system for uniformity in the designs.
We followed a design system to ensure uniformity in the application. I was responsible for creating components in the design system. Our design system was aligned with Apple’s Human Interface guidelines ensuring the color, typography and layouts were in compliance with the guidelines.
I also designed a logo for the application that would represent the app’s purpose.
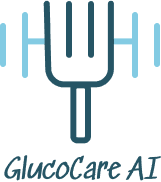
High fidelity design
Designed an interactive and detailed UI with an element of AI in it.
With a solid design system in place, the project moved to high fidelity prototyping, focusing on refining the visuals and interactions. This phase involved designing with a higher level of detail and representing the use of AI in the app.
I designed the onboarding screens, the home screen, the weekly plans screens.
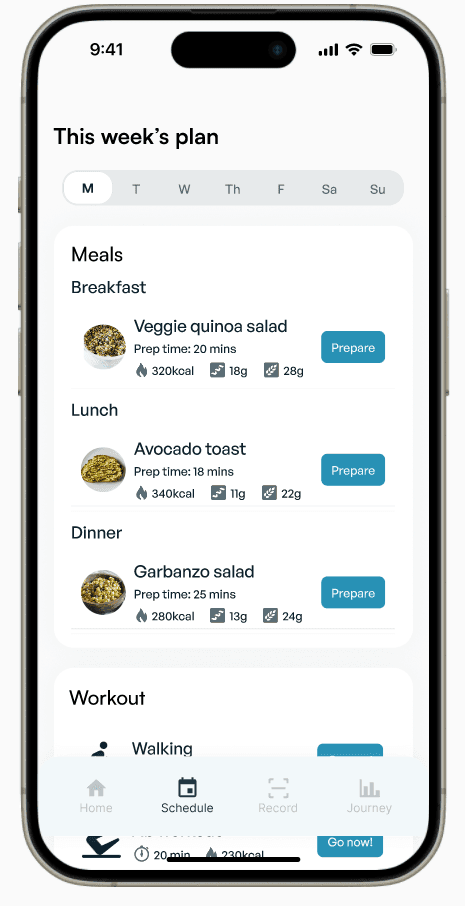
Weekly plans for meals and exercise
Users can plan ahead while buying groceries and planning their days
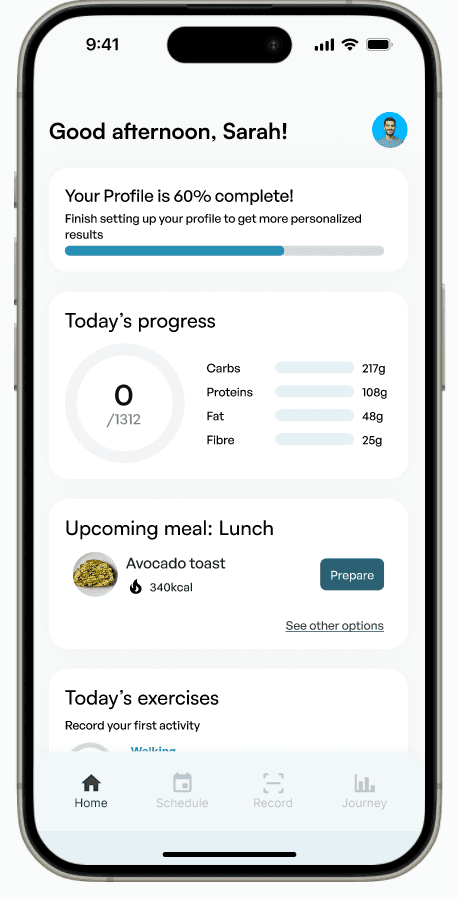
Home screen summarizing daily progress
Users can see current progress and upcoming meals at a glance
User testing
Tested the high fidelity designs with 3 participants via Zoom.
The high fidelity designs were tested with three participants - one pre-diabetic, one diabetic and one non-diabetic. The interviews were conducted via Zoom and the users performed tasks based on the apps functionality. We asked the participants questions after each task to gain feedback.
Positive feedback
The users liked the scanning feature and mentioned that it would be really handy and useful.
The concept of a smart health planner was intriguing for the users and they liked the personalization and smart recommendations.
The participants found the visualization feature motivating and useful.
Areas of improvement
A few design flaws like lack of back button were noted.
A participant expressed their concern when they want to scan food with multiple ingredients that are not separately visible: the system might fail.
Some elements in the UI had issue with visibility and readability.
Redesigning based on the feedback
Redesigned high fidelity screens to accommodate user needs.
Considering the feedback from the users, we redesigned a few aspects of the high fidelity UI by adding some elements like a more engaging AI interaction, allowing users to edit certain aspects while meal tracking, improving the UI visually, etc.
Allow users correct potential mistakes
Fallback for when AI might go wrong - provides users with choice and flexibility.
Additionally, we also added some functionalities like uploading medical reports for more accurate and personalized plans, enhancing feedback mechanisms and increasing AI transparency by providing information about the recommendations
Ensures trust and credibility in AI-suggested plans
Final deliverable
After iterating on the feedback received during testing, changes and improvements, a final prototype was designed.
Impact
Tested the prototype with three users and achieved an overall rating of 9.2/10 for the application’s usability.
On average, users saved 2 hours per day in meal planning and logging activities using the app compared to traditional methods.
Learnings and outcomes
Feedback-based designing
As we tested the prototype with users, we realized how important it was to understand the user’s perspective and modify the app according to user needs.A strong base research goes long way
Since we researched and chose a niche topic, we were able to focus on the target group and their requirements and design for them.Designing for a smaller group poses challenges for testing
Since the app was designed for a specific group of users, it was difficult to test it with real users and get feedback.
To summarize
Designed a meal preparation and logging app for prediabetic patients with an average user satisfaction rating of 9.2/10.
Despite multiple applications for fitness and diabetes management and monitoring, there are no applications focusing on prediabetes. We designed an application focusing on the special group to help them manage prediabetes via lifestyle management. The primary features of the app were food logging using AI scan and providing meal plans and exercises tailored to the user’s preferences and restrictions. We received an average user satisfaction rating of 9.2/10.